Whoops!
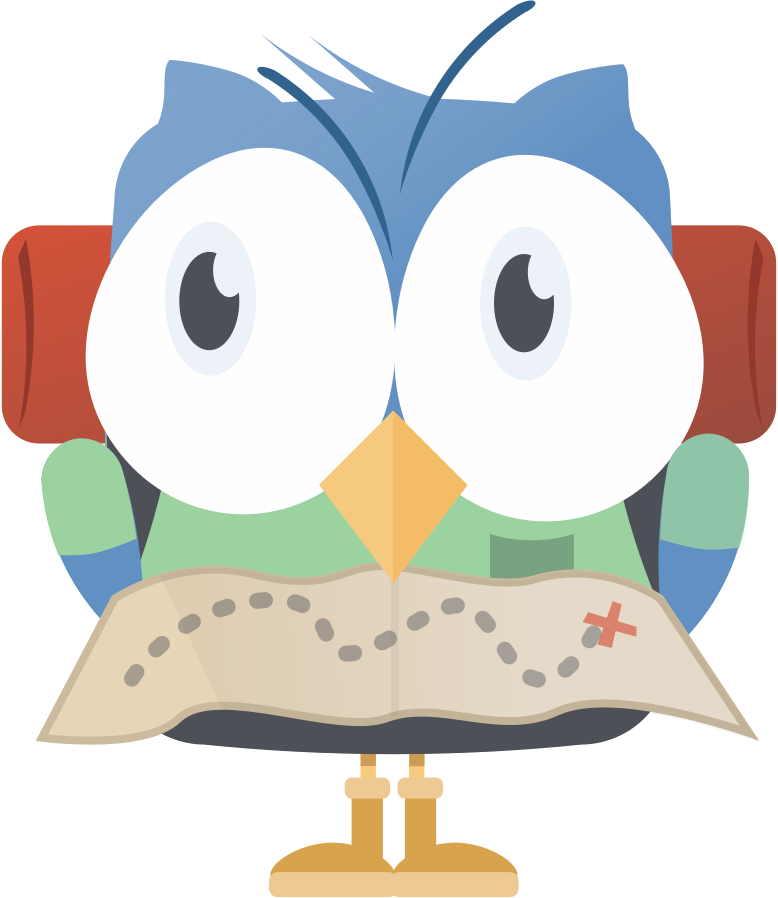
We apologize for the inconvenience, but the page you are trying to find is either out of date or has been removed. Please use the search bar to find what you need, and update your bookmarks. If you don't find what you are looking for, please contact the data.world support team.
Thanks!